Insights
Generative AI for Data Engineering: Pioneering the Future of Data Management - 2024
Introduction
Adding generative artificial intelligence (AI) to the field of data engineering is paving the way for huge changes in this quickly changing field. This technology, which includes tools that can find complicated data patterns and insights, is not just making old ways of doing things better; it’s completely changing them. This blog posts talks about how generative AI is being used in the data engineering lifecycle, the changes it might make, the specific ways it can help data pros, and the careful thoughts it needs.
New Version of the Data Engineering Life Cycle
Usually, the data engineering workflow includes getting data, storing it, processing it, and analyzing it. Generative AI changes this loop by adding features that make many of these tasks automatic and better. AI can now guess and build data infrastructure based on the types and amounts of data that come in, make realistic fake data sets for training models without worrying about privacy, and even dynamically improve data flows to make them more efficient. These improvements not only speed up the processes used to handle data, but they also make them cheaper and easier to expand.
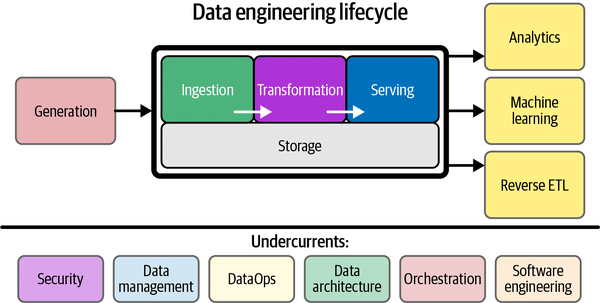
How Generative AI will change data
In data engineering, creative AI has caused a huge amount of change. One of the most important effects is on the quality and quantity of data. Companies can fix the problem of not having enough training data that slows down many machine learning projects by making fake data. Generative AI can also improve data cleaning and prepping, which means that data scientists and engineers don’t have to spend as much time getting data ready. This change not only frees up people, but it also makes it possible to do deeper studies and gain more insights.
Gen AI and the Data Engineer
Generative AI tools are both good and bad for data workers. On the one hand, they provide strong ways to speed up processes, improve data quality, and find new insights that weren’t possible before. But they need to learn new things and get better at things. Data engineers will need to learn how to use AI-based tools to create and manage data, understand new types of data, and add machine learning models to data pipelines. As a result, the data engineer is becoming more like a technologist who not only manages data but also uses AI in a smart way to find its real value.
The Data Side of Gen AI
Adding generative AI to data engineering has a lot of benefits, but it also means that the data itself needs to be looked at more closely. This includes thinking about the ethics of generated data, the flaws that might be built into the data sets, and how reliable AI-generated data is in general. To keep trust and integrity in data-dependent processes and results, it is important to be clear about how data is collected and used.
Data Engineering Lessons to Keep You Safe
When we think about how to use generative AI in data engineering, we should think about what we can learn from both the things that work and the things that don’t. This part talks about some important lessons that can help protect the value and integrity of data. These lessons can help make sure that building data leads to trustworthy and moral outcomes.
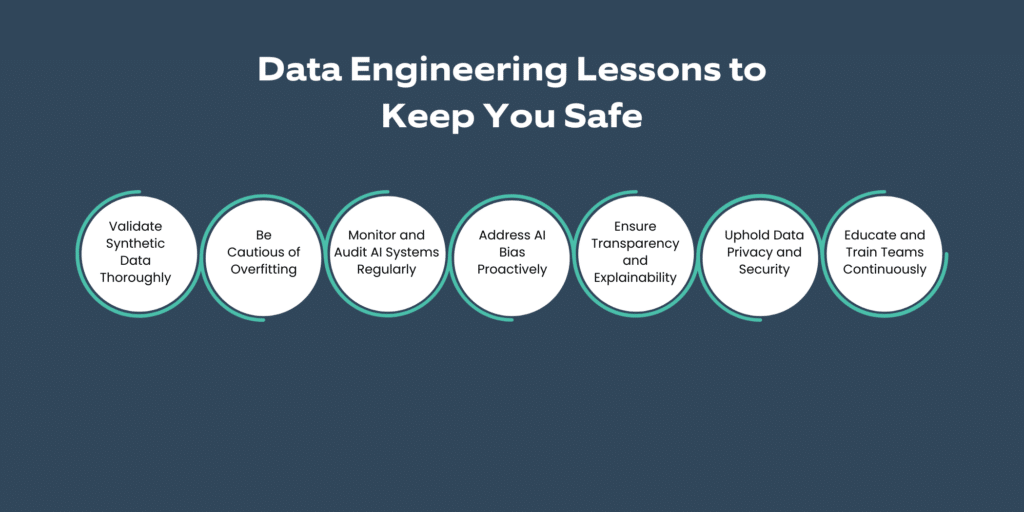
- Validate Synthetic Data Thoroughly
Generated AI can make a lot of fake data, but it is very important that this fake data is just as complicated and variable as real-world data. To make sure that synthetic datasets are correct and useful, data engineers must use strict validation methods. This includes checking for accuracy by comparing fake data to real data and doing a lot of tests to find and fix biases.
- Be Cautious of Overfitting
It’s easy for generative models to overfit, especially ones that were trained on small or very narrow datasets. This can cause models to do well on training data but poorly on data they haven’t seen before. This shouldn’t happen, so data engineers should use cross-validation, regularization, and ensemble methods to make models work better in more situations.
- Monitor and Audit AI Systems Regularly
Continuous monitoring of AI systems is essential to detect any drift in data or model performance over time. Regular audits can help ensure that the systems are functioning as intended and that any deviations are caught and addressed promptly. This is particularly important in regulated industries where data integrity is critical.
- Address AI Bias Proactively
AI systems can unintentionally reinforce or even make biases in the training data worse. Data engineers must constantly look for possible biases and put plans in place to reduce them. As part of a long-term plan to reduce bias, this could mean using a variety of data sources, fairness-aware algorithms, and regular reevaluations of the data and models.
- Ensure Transparency and Explainability
There are times when generative AI models are like “black boxes,” hiding how decisions are made. Aiming for AI models that are clear and easy to understand not only builds trust with partners but also makes it easier to fix problems and make systems better. Some methods that can help make AI processes less mysterious are feature importance scores, model-agnostic explanations, and visualizing decision paths.
- Uphold Data Privacy and Security
Because generative AI can copy and maybe even show private trends in data, keeping data private and safe is very important. To keep data private, data engineers should use the most up-to-date encryption, access controls, and anonymization methods. To stay in compliance and protect sensitive information, strict rules about law and ethics must also be followed.
- Educate and Train Teams Continuously
In order for people who work with creative AI to keep up, their skills must also change. Continuous education and training for data teams can help them understand AI’s strengths and weaknesses better, which can lead to better and more creative use of technology. This schooling shouldn’t just be about technical skills; it should also be about moral issues and how AI applications affect society.
Conclusion
Generative AI is definitely changing data engineering by making it possible to improve, come up with new ideas, and make processes easier to follow. But as this technology gets better, it’s important for workers to not only see its benefits but also be careful about how they use it. Data engineers can lead the way to a more efficient, ethical, and data-driven future by understanding the full range of generative AI’s effects, from its good points to its bad points. As we keep exploring this new area, the data engineer’s job will definitely change. To fully use the power of generative AI in data engineering, they will need to keep learning and adapting.